Background:One popular approach for understanding brain function is by studying electrophysiological recordings from animals. For high-resolution recordings (i.e., the ones using multi-electrode arrays or MEAs), the volume of the recorded neural data becomes significant, which makes it difficult to transmit it wirelessly to the outside world. Thus, neuroscientists currently rely on wired setups to transmit this data, which limit the free movement of the animals. To solve this problem, we recently developed a spike-classification (SC) kernel that performs dimensionality reduction on the sparse neural data by detecting and classifying neuronal spikes. This dimensionality reduction enables the efficient transfer of this information to an external setup for offline analysis.
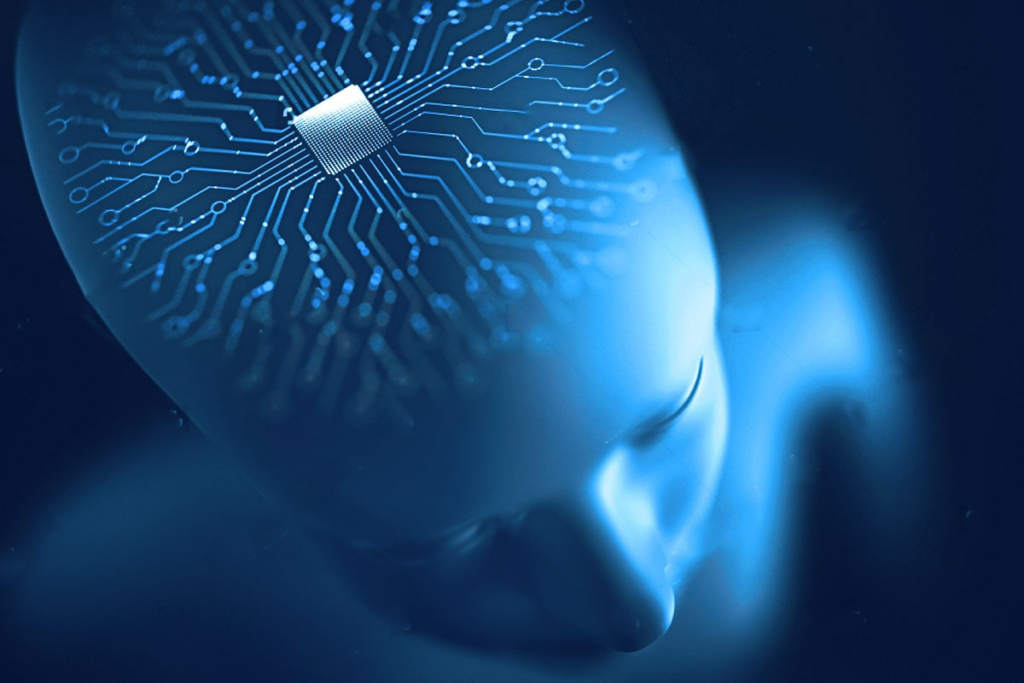
Thesis goal: The goal of this thesis is to port the SC kernel to a memristor-based crossbar (ReRAM) in order to achieve small-form-factor dimensionality reduction for brain-machine interfaces (BMIs).
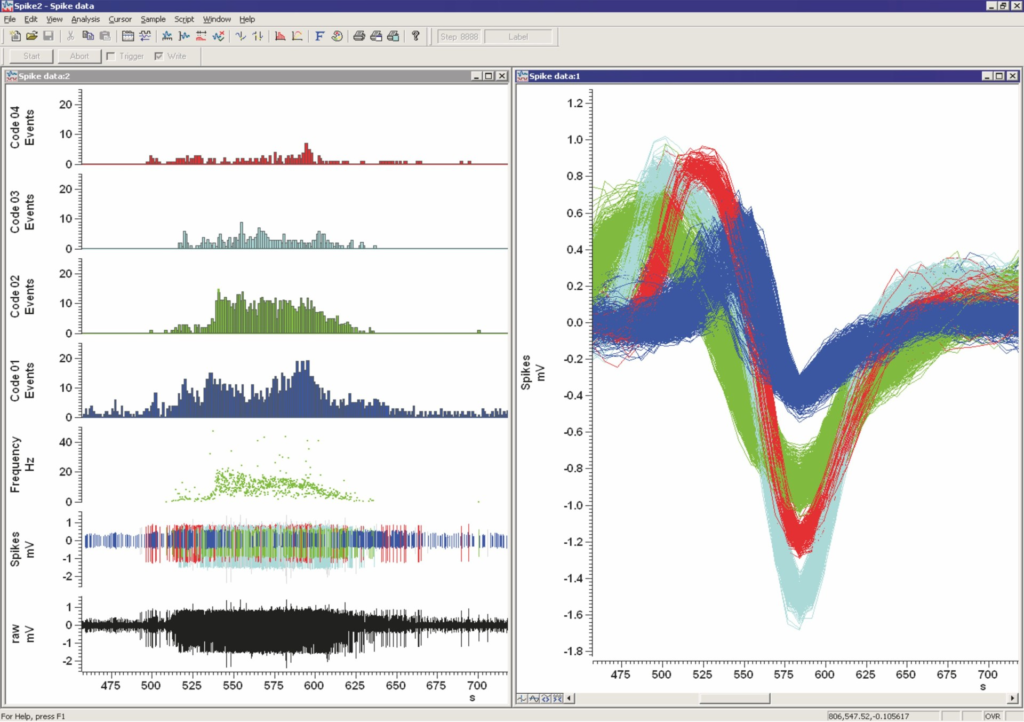
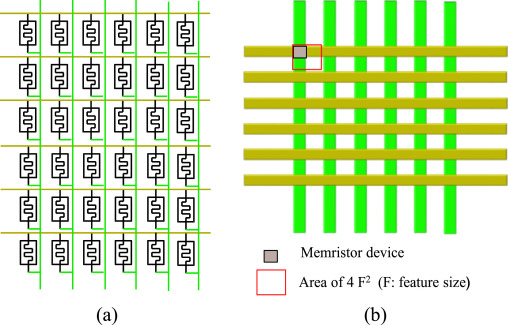
Keywords: Spike classification, memristor, brain-machine interface, deep learning
Prerequisites: Signal processing (basic), machine/deep learning (basic), hardware design
Optionally: Python
Contact: Ali Siddiqi (M.A.Siddiqi@tudelft.nl), Said Hamdioui (S.Hamdioui@tudelft.nl), Christos Strydis (c.strydis@erasmusmc.nl)
Miscellaneous: This is topic offered jointly by the Erasmus Medical Center (Neuroscience department) and the Delft University of Technology (Quantum & Computer Engineering department). It capitalizes on the Convergence between the two universities and offers dual working locations (Rotterdam, Delft), access to extended resources and a truly interdisciplinary environment for conducting research.